Peter Lorenz
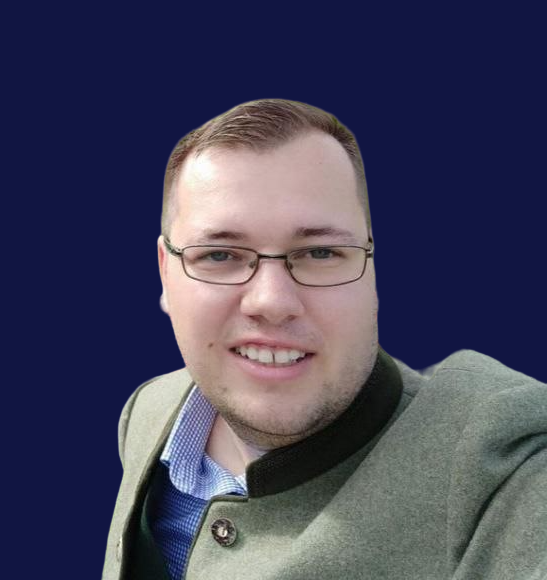
NTU
Machine Learning and Cryptanalysis
I am currently a postdoctoral researcher at Nanyang Technological University (NTU), Singapore, ranked 2nd worldwide in AI research within Thomas Peyrin’s group. My current research conducts adversarial machine learning and cryptanalysis, e.g. model stealing or new adversarial attacks.
Previously, I earned my PhD with “magna cum laude” from Heidelberg University, Germany. Link to PhD thesis.
news
Jan 07, 2025 | Check out my model extraction/stealing - parsed regularly from arxiv! |
---|---|
Mar 18, 2024 | I am happy to announce that I am a reviewer at the CVPR Workshop Robustness of Foundation Models 🎉 |
Jan 29, 2024 | I am accepted for the Oxford Summer School - Representation Learning |
Oct 18, 2023 | I am happy to announce that I am reviewer at ICASSP on the topics federated / split learning and quantum privacy 😄 |
Aug 26, 2023 | Check out my writeups from the Lakera Gandalf hackathon. |
latest posts
Dec 30, 2024 | AdaMSI-FGM |
---|---|
Dec 19, 2024 | AutoAttack |
Nov 29, 2024 | Clear Thinking: Turning Ordinary Moments into Extraordinary Results |